Why is Important to Identify Anomalies in Your Data?
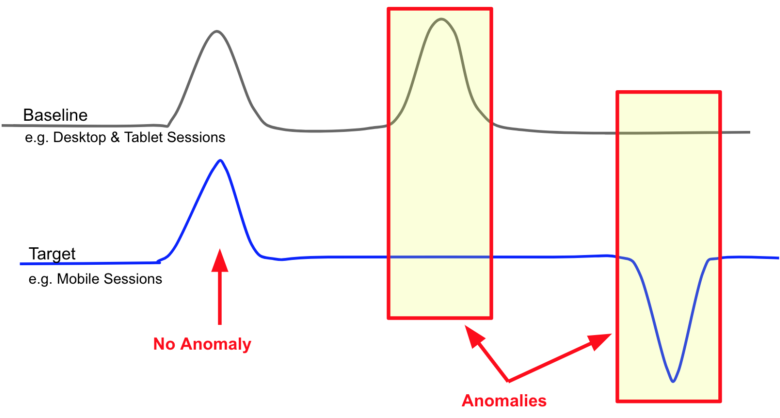
Anomaly detection refers to the process of identifying and removing anomalies from sets of data. It’s sometimes also called outlier analysis and it’s an important process to improve the accuracy and precision of a data set.
Anomaly detection can also be applied to events or observations within a study. When an anomaly is identified, it indicates that there has been an error, whether this is a human error or a technical glitch.
The Bell Curve
When you are presented with a set of data, there will always be an average and a certain part of the data that is more ‘normal’ or ‘expected’ than other parts. There will be fewer data points that lie outside of the average.
Usually, a data set forms a bell curve, where most of the data points sit in the center of the graph, around the median or average data values. There are fewer data points that reside on the edges of the graph, where extreme values are found.
The values that lie outside of the expected threshold are known as anomalies or outliers.
Why is it Important to Identify Outliers?
Data accuracy is essential for any business. When customer and client data is accurate, it can be analyzed properly. Machine learning can use data sets to generate accurate predictions about consumer behavior which can be used by businesses to enhance the customer experience.
Outliers can skew data and make data analysis much harder for businesses. Since most companies rely on their data sets to operate efficiently and retain customers, anomalies can impact business success.
If a business has inaccurate data, the resulting analysis is also inaccurate. This can make it much harder for them to make the right financial and operational decisions.
Anomaly detection is also a vital part of cybersecurity and fraud detection. When extreme values are quickly identified, it keeps data sets safe from hackers.
How Can You Remove Anomalies From Your Data?
When you’re dealing with large data sets, it’s almost impossible to manually scroll through every data set and identify the outliers. Even for small businesses with limited customer and client data, anomaly detection is a time-consuming and labor-intensive task.
Predictive software, such as ServiceNow AIOps, can now be used to identify anomalies quickly and easily in data sets. Machine learning algorithms and anomaly detection algorithms are becoming more and more sophisticated, and they are an efficient way to keep your data as accurate as possible.
Most anomaly detection systems use unsupervised machine learning software that reads large sets of data to identify patterns and detect data points that don’t fit within these recognized patterns.
Machine learning can be used by retailers, banks, manufacturing, and other industries to minimize response times and enable remote monitoring of data. It can run in the background while companies collect more data each day, helping to maintain a constant level of cybersecurity.